| Why Design Research Experiments?
Designing an experiment simply means planning an experiment so that information will be collected that is relevant to the problem under investigation. All too often data collected are of little or no value in an attempted solution to the problem because little or no prior consideration was given to the design of the experiment. Thus, the design of an experiment is the complete sequence of steps taken ahead of time to ensure that the appropriate data will be obtained in a way that permits an objective analysis leading to valid inferences with respect to the stated problem. Important: You should consult with your statistician in the early stages of your proposed research project. He can often recommend a design that is simple and efficient!
Two key considerations in designing an experiment are (i) simplicity and (ii) efficiency. By simplicity, we mean that the simplest experimental design be chosen among many possible candidates to achieve the same proposed objective(s). By efficiency, we mean that the investigation should be conducted as efficiently as possible; that is, every effort should be made to save time, money, personnel and experimental materials.. Fortunately, most simple designs are also efficient (both statistically and economically).
To achieve optimal levels of simplicity and efficiency in designing an experiment, three basic principles should always be considered: replication, randomization and local control (blocking).
Replication means the repetition of treatments in an experiment. There are two reasons why we need replications:
- If a treatment appears only once in an experiment (i.e., n = 1), there is no replication of the treatment and the error associated with the estimate of the treatment effect cannot be estimated. Experimental error occurs when two or more identically treated experimental units fail to yield identical results. Thus, replication of treatments provides an estimate of experimental error;
- Replication also enables us to obtain a more precise estimate of the main effect of any factor since the standard deviation of the mean =
, where represents the true experimental error and n the number of replications.
Randomization legitimatizes the statistical test of significance of observed differences between the treatments. The process of randomization involves random allocation of treatments to the experimental units. Thus the process makes the law of chance applicable to our experimental data and ensures that the data are free from any systematic error. Randomization tends to make experimental errors independent of each other and provides an unbiased estimates of the experimental error and treatment means. Thus, it allows an objective comparison among treatment means.
Local control refers to grouping of the experimental units in such a way that the units within a group (i.e., block) are more homogeneous than are units in different groups. The experimental materials or conditions are more alike within a group. Thus, the variation among experimental units within a group is less than the variation would have been without grouping. This leads to the comparision of treatment effects under more uniform conditions or on the more uniform materials. For example, the total variation in Randomized Complete Block Design (RCBD) is partitioned into variation due to two assignable causes, blocks and treatments, and variation due to a non-assignable cause or experimental error. This latter source of variation is reduced as the variation due to block is removed: Experimental error = Total variation - Treatment variation - Block variation.
Structures of an experimental design
An experimental design consists of two basic structures: Treatment structure and design structure.
- The treatment structure of an experimental design consists of the set of treatments, treatment combinations that the experimenter has selected to study and/or compare. The treatment structure could be a set of t treatments (one-way treatment structure) or a set of treatment combinations (e.g., a two-way factorial arrangement or a higher-order factorial arrangement) plus any controls or other standard treatments.
- The design structure consists of the grouping of the experimental units into homogeneous groups or blocks. Some commonly used design structures are: Completely randomized Design (CRD), Randomized Complete Block Design (RCBD), Latin Square Design (LSD), Incomplete Block Designs (This occurs when the number of treatments exceeds the number of experimental units in a block so that a complete set of treatments cannot occur within each block).
Once the treatment and design structures have been selected, the experimental design is specified by describing exactly the method of randomly assigning (randomizing) the treatments of treatment structure to the experimental units in the design structure. Thus, designing an experiment involves (i) the choice of the treatment structure; (ii) the choice of the design structure, and (iii) the method of randomization.
Experimental Design An Example (Milliken and Johnson 1992, Analysis of Messy Data, p. 55-58)
A nutritionist wants to study the effect of five diets on losing wight. The treatment structure of this experiment is a one-way classification involving five treatments. Many different design structures can be selected:
- If there are 20 'homogeneous' people, then a completely randomized design structure can be used where each diet is randomly assigned to 4 people.
- If there are 10 males and 10 females instead of 20 homogeneous people, then gender of person can be used as a blocking factor; a randomized block design structure could be used, each diet being randomly assigned to two males and two females.
Source | df |
Block (Gender) | 1 |
Diet | 4 |
Error | 14 |
- In some cases, gender of person is not a good choice for a blocking factor because it can also be a type of treatment. In that case, the treatment structure is a two-way factorial arrangement. The design structure would be a completely randomized design.
Source | df |
Gender (G) | 1 |
Diet | 4 |
G * Diet | 4 |
Error | 10 |
- Suppose that the diets have a structure consisting of one standard diet and four new diets made up of the four combinations of two protein levels and two carbohydrate levels. Thus, the treatment structure is a two-way factorial arrangement with a control. The design structure is a completely randomized design.
Source | df |
Gender (G) | 1 |
Diet | 4 |
Control vs. 22 (A) | 1 |
Protein (P) | 1 |
Cabohydrate(C) | 1 |
P * C | 1 |
G * Diet | 4 |
G * A | 1 |
G * P | 1 |
G * C | 1 |
G * P * C | 1 |
Error | 10 |
Identifying Sizes of Experimental Units
While it has been repeatedly emphasized that the statistician should be consulted in designing research experiments, he does not always get the chance to design an experiment. Instead, when he is presented with the experimental data, he must identify the type of experimental design that the experimenter has employed. One important step in the identification process is to determine if more than one size of experimental unit has been used.
The experimental designs that have several sizes of experimental units (SSEU designs) have two important characteristics:
- The treatments consist of at least a two-way set of treatment combinations and the design structure consists of incomplete blocks;
- More than one size of experimental unit is used in the experiment with a SSEU design.
Some commonly used SSEU designs are: split-plot type designs, repeated measures designs and some nested type designs. The feature that distinguishes split-plot designs from repeated measures designs is that the levels of the treatments can be applied to the various sizes of experimental units by using randomization; in contrast, repeated measures designs involve a step where the levels of at least one treatment (usually time) cannot be assigned at random. Nesting occurs most often in the design structure of an experiment, where smaller experimental units are nested within a large size if they are different for each large experimental unit.
SSEU Design: An example (Milliken and Johnson, Analysis of Messy Data, p. 77-80)
A meat scientist wants to study the effect of three levels of temperature (T), two types of packaging (P), four types of lighting (L) and four levels of light intensity (I) on the color of meat stored in a meat cooler for seven days. Six cooler are avilable for the experiment, and the three temperatures (34 oF, 40 oF and 46 oF) are each randomly to two coolers. Each cooler is partitioned into 16 compartments an a 4 x 4 grid. Because the light intensities are regulated by distance, all partitions in a column are assigned the same intensity. The types of light are randomly assigned to each partition within a column. The following figure depicts assignment of the various treatments to the 16 compartments in each cooler:
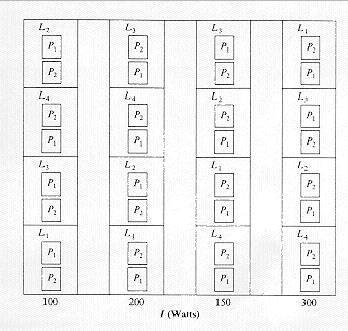
The important first step towards analyzing this experiment is to identify the different sizes of experimental units. This is a split-split-split-plot experiment consisting of four sizes of experimental units. The linear for this design can be described as follows,
yijklmn = | µ + Ti + aij | Cooler (temperature) |
 | + Ik + (TI)ik + bijk | Column (light intensity) |
 | + Lm + (TL)im + (IL)km + (TIL)ikm+ cijkm | Partition (type of light) |
 | + Pn + (TP)in + (IP)kn + (TIP)ikn + (LP)mn + (TLP)imn + (ILP)kmn + (TILP)ikmn + dijkmn | Half-partition (packaging) |
Analysis of variance table for meat in cooler example
Source of variation | Degrees of freedom |
Cooler (temperature) analysis |  |
T | 3-1 = 2 |
Error a = Cooler (temperature) | 3(2-1) = 3 |
 |  |
Column (light intensity) analysis |  |
I | 4-1 = 3 |
T*I | (3-1)*(4-1) = 6 |
Error b = I*Cooler(temperature) | (4-1)*3(2-1) = 9 |
 |  |
Partition (type of light) analysis |  |
L | 4-1 = 3 |
L*T | (4-1)*(3-1) = 6 |
L*I | (4-1)*(4-1) = 9 |
L**I*T | (4-1)*(4-1)*(3-1) = 18 |
Error c = L(I)*Cooler(temperature) | 4(4-1)*3(2-1) = 36 |
 |  |
Half-partition (packaging) analysis |  |
P | 2-1 = 1 |
P*T | (2-1)*(3-1) = 2 |
P*I | (2-1)*(4-1) = 3 |
P*I*T | (2-1)*(4-1)*(3-1) = 6 |
P*L | (2-1)*(4-1) = 3 |
P*L*T | (2-1)*(4-1)*(3-1) = 6 |
P*L*I | (2-1)*(4-1)*(4-1) = 9 |
P*L*I*T | (2-1)*(4-1)*(4-1)*(3-1) = 18 |
Error d = P(L*I)*Cooler(temperature) | 4*4*(2-1)*3(2-1) = 48 |
Total | 3*2*4*4*2-1 = 191 |
SSEU Design: Example Two (Milliken and Johnson, Analysis of Messy Data, p. 80-84)
A veterinarian has two techniques of fusing the joint of a horse's foot after it is broken, and she wants to determine if one technique is better than the other. The experiment consists of taking four horses, breaking a joint on each horse, repairing it with one of the two techniques, and determining the strength of the fused joint four months later. She also wants to determine if the same techniques work equally well for front feet and back feet. Thus, her plan is to break the joint on a front foot, wait until it heals, and then break the joint on a rear foot. What would be an appropriate experimental design for her experiment?
This experiment consists of two different sizes of experimental units: The feet are the smaller experimental units while the horses are the larger experimental units. The treatment structure of this experiment is a 2 (techniques) x 2 (positions) x 2 (two times) factorial. The design structure is an incomplete block design where each horse is a block and there are two observations per block (horse). Since the blocks are incomplete, some of the treatment structure information will be confounded with block or horse effects. There are various ways of assigning the treatment combinations to the two feet of a horse. Since the primary objective of this experiment is to compare the two fusion techniques, the following design yields a fusion comparison that is not confounded with horses and thus achieves the design goal of having the most important effect being compared on the smaller experimental units (feet).
Horse 1 | Horse 2 | Horse 3 | Horse 4 |
F1 P1 T1 | F2 P1 T1 | F1 P1 T2 | F2 P1 T2 |
F2 P2 T2 | F1 P2 T2 | F2 P2 T1 | F1 P2 T1 |
The two fusion techniques are F1 and F2, the two times are T1 and T2, and the two positions are P1 and P2.
ANOVA Table for the Fusion Experiment
Source of variation | Df |
Between-horse |  |
F*P | 1 |
F*T | 1 |
P*T | 1 |
Error (Horse) | 4 |
 |  |
Within-horse |  |
F | 1 |
T | 1 |
P | 1 |
F*P*T | 1 |
Error (Foot) | 4 |
|
|